What is Open-RAN and How ML Plays a Big Role in its Development?
In this post we will be talking about the following topics: What is Open RAN? Open RAN and 5G. Current challenges and its limitations. The importance of AI/ML for Open-RAN. Open RAN vs Legacy network architecture To start talking about open-RAN we can first have a look of how a traditional network architecture is designed:…
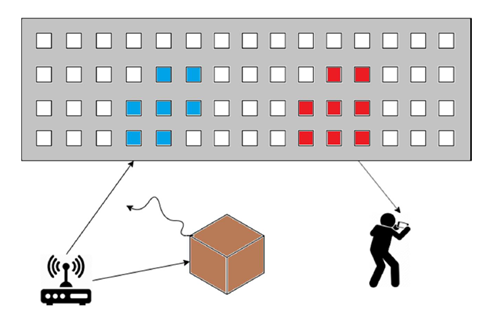
Beyond mMIMO Large-scale Wireless Sensing
The aim of this post is to describe the work “Assessing Wireless Sensing Potential with Large Intelligent Surface” published in IEEE Open Journal of the Communications Society. I will try to show the main ideas behind it in a more simplified manner. Please note that for a more detailed description of the methods and the…
Slotted ALOHA with Reinforcement Learning
In this article we will discuss the potential of reinforcement learning (RL) to learn a backoff control policy for slotted ALOHA-type random access. We will use deep reinforcement learning (DRL) to learn a policy for multi user random access system. Slotted ALOHA Background Slotted ALOHA (sALOHA) protocol [1] for random access in wireless networks has…
Emergence of MAC Protocols with Multi-Agent Reinforcement Learning
In this post we will discuss how can we allow medium access control (MAC) protocols to emerge with multi-agent reinforcement learning (MARL). Current MAC protocols are designed by engineers as a predefined set of rules, but have we addressed the question of what happens if we let the network come up with its own MAC…
Optimizing On-demand Slices Selection in Core Networks
The evolution of wireless core networks provides a flexible framework for network traffic management. The most famous concept is network slicing. In this article an on-demand network slices selection problem in the core network will be introduced in section 1, and the optimization formulation of the slices selection problem will be defined in section 2….
Neural Autoregressive Models and Normalizing Flows
Estimation of probability density of high dimensional complex data distributions is a long-standing challenge in many different fields, including machine learning. Neural Autoregressive Density Estimation (NADE) is a method that estimates data distributions by modeling each term in the probability chain rule with a parameterized function [1]. (1) The indices in (1) represent an…