In this article we will discuss the potential of reinforcement learning (RL) to learn a backoff control policy for slotted ALOHA-type random access. We will use deep reinforcement learning (DRL) to learn a policy for multi user random access system.
Slotted ALOHA Background
Slotted ALOHA (sALOHA) protocol [1] for random access in wireless networks has been around for over 50 years. Thanks to its simple design and configuration it is still a topic of great interest, specifically in the machine-type communication (MTC) paradigm in 5G and beyond communication systems. In sALOHA users in the network randomly transmit in a grant-free manner with the hope that the receiver will decode their packets. For instance, consider a network of N = 10 sensor nodes in a certain geographic area and a central unit that collects the information from these sensors/users. Each sensor reports its readings to the central unit in form of packets containing the information about its reading. Whenever a sensor has some reading during the day it transmits its packet without coordinating with other sensors and without being scheduled by the central unit. In sALOHA we divide time in slots and enforce each sensor through synchronization to always transmit at the beginning of each time slot.
However, what happens when two or more sensors transmit at the same time? This is where it becomes complicated. Their packets collide and the transmission of every user fails. They will have to send their packet again. Let us assume that each of the 5 users have a packet to transmit and they transmit them with a certain transmit probability pn. Now having the same pn for all the users will lead to what we call unstable behavior, i.e., eventually no user is able to transmit its packet, and the performance goes to zero. The performance is commonly measured with the throughput, i.e., the number of successful transmissions (packets) in a given time. To tackle this issue certain backoff techniques have been proposed in the literature, i.e., using some kind of feedback signal from the receiver to indicate whether the transmission on the channel resulted in a collision or not. There are other feedback signals considered in the literature, such as success/no-success and ternary feedback for success, collision, idle events, but in this work we only consider collision/no-collision feedback. If a collision event happens receiver sends 0 bit as feedback, and if no-collision event happens 1 bit is sent by the receiver. The exponential backoff (EB) is a commonly used backoff technique in which users reduce their transmit probability exponentially for each collision event. For a non-collision event they transmit with a maximum probability pmax and usually it is set to pmax = 1. The protocol is still distributed, i.e., each user independently calculates its pn after each collision or no-collision event. In binary exponential backoff (BEB) [2] the backoff factor is set to 2, i.e., after i consecutive collisions, the probability of a user is reduced to pn*2-i. The theoretical throughput for sALOHA is 1/e.
This EB technique introduced another issue. It had been observed in the simulation experiments that the overall throughput of the network was very large, i.e., 0.6 or 0.7, compared to theoretical results that is 1/e = 0.36. It took researchers some time to figure out the reason for this behavior. It is commonly referred to as the capture effect. With pmax = 1 a user would capture the channel for some time, while starving other users, resulting in high overall throughput, but causing unfairness in the distribution of resources among users. Therefore, it is important for a protocol not only to have a good throughput but also to be fair.
Motivation
Since then there has been a tremendous amount of work on sALOHA. However, there has been no consensus on the performance and this throughout-fairness tradeoff. In fact, due to underlying assumptions of different models considered, the results are sometimes contradictory and confusing [4]. For instance, there is no consensus what is the optimal backoff factor or what kind of backoff technique is good. What is a good model for traffic arrival process? Moreover, most of the models assume that each user always has a packet to transmit, which is not true for MTC applications where the traffic is sporadic. This complexity of the process motivates us to analyse the performance of sALOHA with reinforcement learning and to learn a backoff protocol (a policy) using the simple aforementioned binary feedback signal.
Problem Formulation with RL
In RL an agent in a state S interacts with an environment without knowing the dynamics of the environment by choosing an action from a pre-defined action space, receives a reward about how good the action was and moves to the next state S. In this case, the environment is the random access channel, action is to TRANSMIT (1) or NOT TRANSMIT (0), and we define reward as SUCCESS. If a packet has been successfully transmitted over the channel, the reward is 1, and it is 0 otherwise. The environment is show in the Fig. 1. For multiple number of users, every user is an RL agent. The objective of the agent is to maximize the reward in the long run. We assume homogeneous users, and we train them in a centralized way using neural network (DQN algorithm [5]) in such a way that they all learn the same policy that is implemented in a distributed manner for decision making. The purpose of this work is to find a policy for ALOHA-type random access, that provides us better throughput as well as fairness among users.
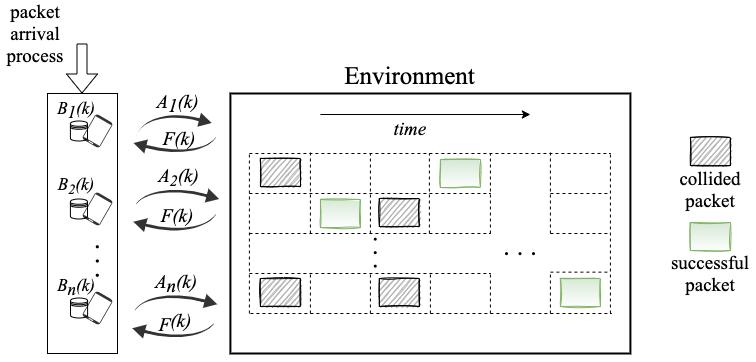
For this purpose, each user uses its local observation to decide whether to transmit or not. The local observations make the state of the user. For time slot k the state contains previous action, previous feedback and buffer state. Buffer state indicates whether a user has a packet in its queue or not. The state of a user at time k can be written as:
Sn(k) = [An(k-1), F(k-1), Bn(k-1)] ——— (1)
Where An(k) ∈ {0, 1}, and F(k) ∈ {0, 1}, Bn(k) ∈ {0, 1}, which gives us total 23 states. Naturally, when there is no packet in the buffer of user n, i.e., Bn(k) = 0, the transmit probability is set to zero. Therefore, we are only interested in 4 states when Bn(k) = 1.
Procedure
At each time slot k new packet arrives using the Poisson arrival process with average arrival rate λ. We assume that each user can only contain maximum of one packet. If there is a packet in user’s buffer, it follows the learned policy to decide whether to transmit or not. The output of the DQN is the Q-values corresponding each action. These Q-values are used to calculate the transmit probability with softmax policy. After taking action all users receive a common feedback signal F(k) and use this to calculate the reward. The reward is global, i.e., same for all users. If the transmission was successful, every user receives reward that equals 1 or 0 otherwise.
Simulation Results
In Eq. 1 we have seen that there are only 4 possible states for each user, and it is interesting to see how the transmit probability changes for each state as we increase the arrival rate from 0.2 to 2 as shown in Fig. 2. We use transfer learning to train from lower to higher arrival rates. Learning for a small state space allows us to look into the details of how the policy changes for different arrival rates and helps us visualize it.
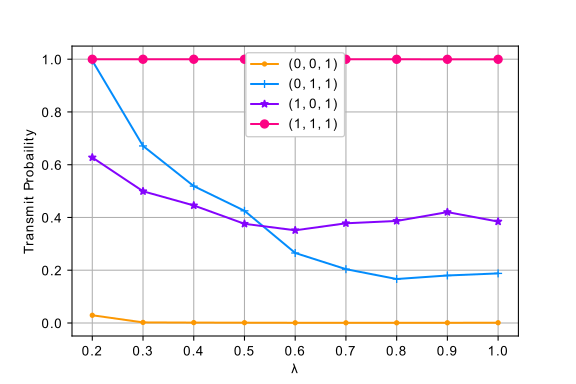
We analyse the performance of this DQN-based random access (DQN-RA) protocol for throughput and fairness. For fairness we propose to use a parameter we refer to as age of packet (AoP). Popular Jain’s fairness index doesn’t consider short-term fairness, and AoP can measure fairness as well indicate the packet delay. If user transmits a packet, AoP(k) = 0; otherwise the AoP increases linearly with time, i.e., AoP(k) = AoP(k-1) +1 . We compare the performance of DQN-RA with BEB for 10 users. Clearly the proposed algorithm outperforms BEB in terms of both throughput and fairness. We compare the performance of DQN-RA with two types of EB, and we divide them into symmetric EB (SEB) and non-symmetric EB (nSEB). nSEB is the same as mentioned above, where each user changes its transmit probability based on whether there was collision or not, but in case of no-collision, pn = pmax. On the other hand, in SEB every user increases or decreases the pn exponentially if no-collision or collision event happens respectively. SEB is a more fair scheme, because all the users change their probabilities. We compare for two backoff factors, binary (B) and 1.25 with the DQN-RA.
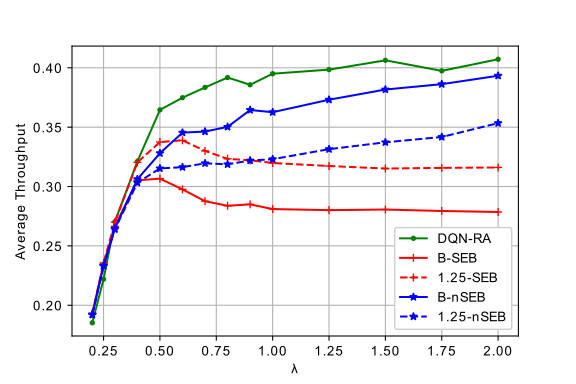
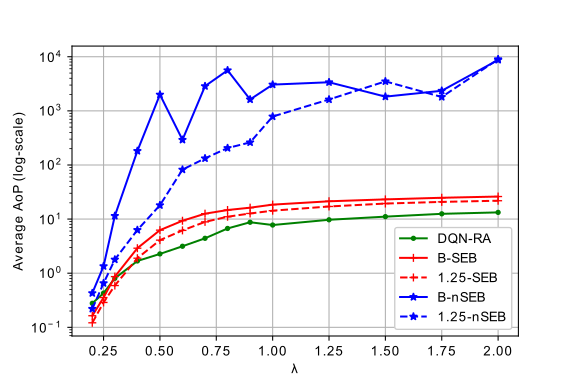
Surprisingly, DQN-RA is inherently fair among users compared to the EB without explicitly optimizing it for fairness. Moreover, even though the results show average AoP for fairness over 10 users it is interesting to see the AoP of individual users, and we have observed (not shown here) that BEB is quite unfair due to capture effect. DQN-RA provides us a balanced trade-off between throughput and fairness compared to EB schemes.
For the future of this topic it would be interesting to see if adding more past feedback will improve the performance or not. Moreover, how does this scheme behaves if the number of users is large?
References
- N. Abramson, “The throughput of packet broadcasting channels,” IEEETransactions on Communications, vol. 25, no. 1, pp. 117–128, 1977.
- B.-J. Kwak, N.-O. Song, and L. Miller, “Performance analysis of exponential backoff,” IEEE/ACM Transactions on Networking, vol. 13,no. 2, pp. 343–355, 2005.
- . L. Massey, “Some new approaches to random-access communication,”in Proceedings of the 12th IFIP WG 7.3 International Symposium on Computer Performance Modelling, Measurement and Evaluation,Performance ’87, (NLD), p. 551–569, North-Holland Publishing Co.,1987.
- L. Barletta, F. Borgonovo, and I. Filippini, “The throughput and access delay of slotted-aloha with exponential backoff,” IEEE/ACM Transactions on Networking, vol. 26, no. 1, pp. 451–464, 2018.
- V. Mnih et al., “Human-level control through deep reinforcement learning,” Nature, vol. 518, no. 7540, 2015.
cheap retro jordans
$ 73.1Cheap jordans 11 Low GS Citrus For Women
cheap louis vuitton Original vernis handbags
louis vuitton epi ivory
ugg bags for sale
Therefore, in this GG Marmont bag series, you will also feel the retro style. In fact, it also carries the nostalgia of designer Alessandro Michele. At the same time, it also achieves the integration of art and fashion, and continuous innovative design…
louis vuitton speedy bag
Hermes’ Birkin bags have always been pricey, but their alligator and crocodile skin versions can cost upwards of $100,000.
cheap louis vuitton Original handbags outlet online
Described by the brand as ‘pure luxury’, it is crafted from rare foreign crocodile leather, with braided handles and suede-lined compartments. The leather version is only $3,450.
https://images.google.co.uk/url?q=https://www.topuggbootsoutlet.com/
|One thing you are going to want to do is always keep an eye open for changes in style. Styles are constantly changing, and you can find out what is new by looking at fashion magazines every now and then. They are likely going to showcase the new trend…
tasche von louis vuitton
When capital intervenes, the process of industrialization is endowed with legendary stories of brand history, and “luxury goods” that are accessible to the general public are born, what can buying big brands support? Some use it as armor, while other…
http://www.torrent.ai/lt/redirect.php?url=https://www.toplouisvuittonoutlet.com/
|Subscribe to a fashion newsletter of some sort so that you are up to date with some of the latest fashion trends. When you do that, you’ll be ready for every season and you will be first in your social group to have the latest fashions.
cheap louis vuitton bags for women
Models walked in flight jackets, bravely carrying music accents and rushing into the play, you know it’s coming again.
louis vuitton suhali
Generally speaking, crocodile skin, alligator skin and snake skin are unique and precious leather materials that Cheap authentic Original Women louis Vuitton Sale|Authentic Women louis Vuitton Sale|Cheap Louis Vuitton Handbags Factory Outlet Online Sal…
https://cse.google.dz/url?sa=i&url=https://www.topchristianlouboutinoutlet.com/
|There are hundreds of thousands of hair accessory possibilities. You can choose from lots of options, including headbands, scrunchies, bows, barrettes and extensions. Include various hair accessories for practical and aesthetic purposes. The right hai…
cheap louis vuitton luggage sets
This popular Cheap authentic Original Women louis Vuitton Sale|Authentic Women louis Vuitton Sale|Cheap Louis Vuitton Handbags Factory Outlet Online Sales sale online store ,LV classic bag DAUPHINE is equipped with special rare materials. The biggest f…
camiseta miami heat 2020
|Avoid any horizontal stripes if your weight is higher. This puts emphasis on how wide your body is, making it look even wider. Instead, pick a pattern that is linear or vertical which can make you look thinner.
https://www.toplouisvuittonoutlet.com/product_cat-sitemap1.xml
|Subscribe to a fashion newsletter of some sort so that you are up to date with some of the latest fashion trends. When you do that, you’ll be ready for every season and you will be first in your social group to have the latest fashions.
https://www.google.com.my/url?sa=t&url=https://www.camisetaslakers.es/
|Be careful when using mascara, and do try getting more product on the brush by pushing it carefully into the container. It will not get more product on the brush, and will trap air inside the bottle. This ups the odds of bacteria growing in it. Move y…
https://images.google.ch/url?sa=t&url=https://www.camisetasceltics.es/
|On a hot summer’s day, wearing your hair up can be fashionable and functional. Long, loose hair can get in the way during work or play. If you do not have time for a more elaborate style, just pull it into a cute bun.
buy cialis tadalafil
Slotted ALOHA with Reinforcement Learning – Knowledge Sharing Platform
help with college essays
Slotted ALOHA with Reinforcement Learning – Knowledge Sharing Platform
cheap custom essays online
Slotted ALOHA with Reinforcement Learning – Knowledge Sharing Platform
write my law essay
Slotted ALOHA with Reinforcement Learning – Knowledge Sharing Platform
write my essay fast
Slotted ALOHA with Reinforcement Learning – Knowledge Sharing Platform
cheap essay writers
Slotted ALOHA with Reinforcement Learning – Knowledge Sharing Platform
persuasive essay helper
Slotted ALOHA with Reinforcement Learning – Knowledge Sharing Platform
english essay writing service
Slotted ALOHA with Reinforcement Learning – Knowledge Sharing Platform
professional essay help
Slotted ALOHA with Reinforcement Learning – Knowledge Sharing Platform
essay customer service
Slotted ALOHA with Reinforcement Learning – Knowledge Sharing Platform
best online essay writers
Slotted ALOHA with Reinforcement Learning – Knowledge Sharing Platform
essay writer helper
Slotted ALOHA with Reinforcement Learning – Knowledge Sharing Platform
what can i write my essay on
Slotted ALOHA with Reinforcement Learning – Knowledge Sharing Platform
essay on helping others
Slotted ALOHA with Reinforcement Learning – Knowledge Sharing Platform
admission essay writing service
Slotted ALOHA with Reinforcement Learning – Knowledge Sharing Platform
essay writing help for high school students
Slotted ALOHA with Reinforcement Learning – Knowledge Sharing Platform
magellan rx specialty pharmacy
Slotted ALOHA with Reinforcement Learning – Knowledge Sharing Platform
your rx pharmacy grapevine tx
Slotted ALOHA with Reinforcement Learning – Knowledge Sharing Platform
online pharmacy ultram
Slotted ALOHA with Reinforcement Learning – Knowledge Sharing Platform
buying percocet online pharmacy
Slotted ALOHA with Reinforcement Learning – Knowledge Sharing Platform
asda pharmacy viagra prices
asda pharmacy viagra prices
cialis no prescription paypal
cialis no prescription paypal
female viagra india
female viagra india
cialis free shipping canadian
cialis free shipping canadian
best place to buy cialis
best place to buy cialis
viagra price comparison uk
viagra price comparison uk
buy viagra pharmacy uk
buy viagra pharmacy uk
viagra tablets australia
viagra tablets australia
receive cialis overnight
receive cialis overnight
buy cheap viagra
buy cheap viagra
sildenafil tablets 100 mg
sildenafil tablets 100 mg
tadalafil bulk powder
tadalafil bulk powder
cialis ebay
cialis ebay
cialis online usa
cialis online usa
solutions rx pharmacy
solutions rx pharmacy
generic viagra online pharmacy reviews
generic viagra online pharmacy reviews
over the counter sildenafil
over the counter sildenafil
canada cialis online
canada cialis online
best price for sildenafil 50 mg
best price for sildenafil 50 mg
cialis super active vs cialis
cialis super active vs cialis
printable cialis coupon
printable cialis coupon
indian pharmacy accutane
indian pharmacy accutane
viagra 25 mg coupon
viagra 25 mg coupon
buy viagra online cheap
buy viagra online cheap
generic sildenafil citrate 100mg
generic sildenafil citrate 100mg
ordering sildenafil online without prescription
ordering sildenafil online without prescription
order viagra pills online
order viagra pills online
cheap sildenafil
cheap sildenafil
cialis san diego
cialis san diego
tadalafil 5mg generic from us
tadalafil 5mg generic from us
buy cialis online without prescription
buy cialis online without prescription
cialis daily cost
cialis daily cost
bactrim sweating
bactrim sweating
metronidazole paralysis
metronidazole paralysis
gabapentin cessation
gabapentin cessation
valacyclovir transmission
valacyclovir transmission
nolvadex ervaringen
nolvadex ervaringen
natural alternative to lyrica
natural alternative to lyrica
furosemide lymphedema
furosemide lymphedema
furosemide lisinopril
furosemide lisinopril
metformin hcl850mg
metformin hcl850mg
long term side effects of rybelsus
long term side effects of rybelsus
rybelsus what does it do
rybelsus what does it do
rybelsus price without insurance
rybelsus price without insurance
when to increase zoloft dosage
when to increase zoloft dosage
cephalexin alcohol
cephalexin alcohol
flagyl protostat
flagyl protostat
can fluoxetine and clonazepam be taken together
can fluoxetine and clonazepam be taken together
gabapentin cymbalta
gabapentin cymbalta
how much is lexapro without insurance
how much is lexapro without insurance
duloxetine sexual side effects
duloxetine sexual side effects
escitalopram not generic name
escitalopram not generic name
gabapentin r665
gabapentin r665
viagra super active 100mg
viagra super active 100mg
can keflex cause yeast infection
can keflex cause yeast infection
cephalexin acne
cephalexin acne
does ciprofloxacin treat sinus infection
does ciprofloxacin treat sinus infection
can bactrim treat bacterial vag
can bactrim treat bacterial vag
how long does it take for bactrim to get out of your system
how long does it take for bactrim to get out of your system
amoxicillin and ibuprofen
amoxicillin and ibuprofen
citalopram tinnitus went away
citalopram tinnitus went away
diclofenac sodium topical gel 1%
diclofenac sodium topical gel 1%
ddavp nasal drops dogs
ddavp nasal drops dogs
cozaar manufacturer coupon
cozaar manufacturer coupon
wellbutrin vs effexor
wellbutrin vs effexor
diltiazem cd 240mg
diltiazem cd 240mg
ezetimibe ameliorates metabolic disorders and microalbuminuria in patients with hypercholesterolemia
ezetimibe ameliorates metabolic disorders and microalbuminuria in patients with hypercholesterolemia
goodrx contrave
goodrx contrave
flomax products livermore ca
flomax products livermore ca
flexeril and pregnancy
flexeril and pregnancy
side effects of depakote
side effects of depakote
augmentin antibiotic
augmentin antibiotic
amitriptyline dosage
amitriptyline dosage
is aripiprazole a controlled substance
is aripiprazole a controlled substance
how long does it take for allopurinol to work
how long does it take for allopurinol to work
aspirin vs tylenol
aspirin vs tylenol
what is the drug baclofen
what is the drug baclofen
bupropion withdrawal timeline
bupropion withdrawal timeline
celexa lexapro
celexa lexapro
celecoxib adverse effects
celecoxib adverse effects
how long does buspar last
how long does buspar last
augmentin while breastfeeding
augmentin while breastfeeding
can celebrex cause weight gain
can celebrex cause weight gain
can abilify cause weight gain
can abilify cause weight gain
acarbose india
acarbose india
ionization lipophilicity and solubility properties of repaglinide
ionization lipophilicity and solubility properties of repaglinide
robaxin and tramadol
robaxin and tramadol
protonix 40 mg
protonix 40 mg
50 units of semaglutide is how many mg
50 units of semaglutide is how many mg
actos-leipzig.de
actos-leipzig.de
side effects of remeron at 15 mg
side effects of remeron at 15 mg
methocarbamol vs tizanidine
methocarbamol vs tizanidine
synthroid pharmacodynamics
synthroid pharmacodynamics
stromectol online
stromectol online
venlafaxine dosage 300 mg side effects
venlafaxine dosage 300 mg side effects
can you take tamsulosin and finasteride together
can you take tamsulosin and finasteride together
reviews on spironolactone
reviews on spironolactone
sitagliptin nitrosamine impurity
sitagliptin nitrosamine impurity
voltaren gel at costco
voltaren gel at costco
online pharmacy xanax cheap
online pharmacy xanax cheap
cialis online pills
cialis online pills
sildenafil for pe
sildenafil for pe
sildenafil coupons
sildenafil coupons
levitra kaufen
levitra kaufen
peptide liquid tadalafil
peptide liquid tadalafil
online pharmacy hydrocodone
online pharmacy hydrocodone
buy levitra without a prescription
buy levitra without a prescription
stromectol cream
stromectol cream
ivermectin 6mg dosage
ivermectin 6mg dosage
stromectol 3mg tablets
stromectol 3mg tablets
buy ivermectin cream for humans
buy ivermectin cream for humans
erectile dysfunction vardenafil
erectile dysfunction vardenafil
ivermectin 3mg tablets
ivermectin 3mg tablets
tadalafil patent
tadalafil patent
vardenafil hcl canada
vardenafil hcl canada
buy generic viagra in india
buy generic viagra in india
paypal cialis tadalafil
paypal cialis tadalafil
otc female viagra
otc female viagra
buy stromectol pills
buy stromectol pills
what is gabapentin for dogs
what is gabapentin for dogs
does prednisone make you pee
does prednisone make you pee
trimox drug information
trimox drug information
amoxicillin for sore throat
amoxicillin for sore throat
does trazodone show up on a drug test
does trazodone show up on a drug test
purchase provigil in mexico
purchase provigil in mexico
side effects of cipro
side effects of cipro
can i drink on cephalexin
can i drink on cephalexin
is lisinopril 10 mg a low dose
is lisinopril 10 mg a low dose
valtrex and covid
valtrex and covid
metformin er side effects
metformin er side effects
doxycycline for std
doxycycline for std
keflex generation
keflex generation
lyrica generic names
lyrica generic names
can tamoxifen cause cancer
can tamoxifen cause cancer