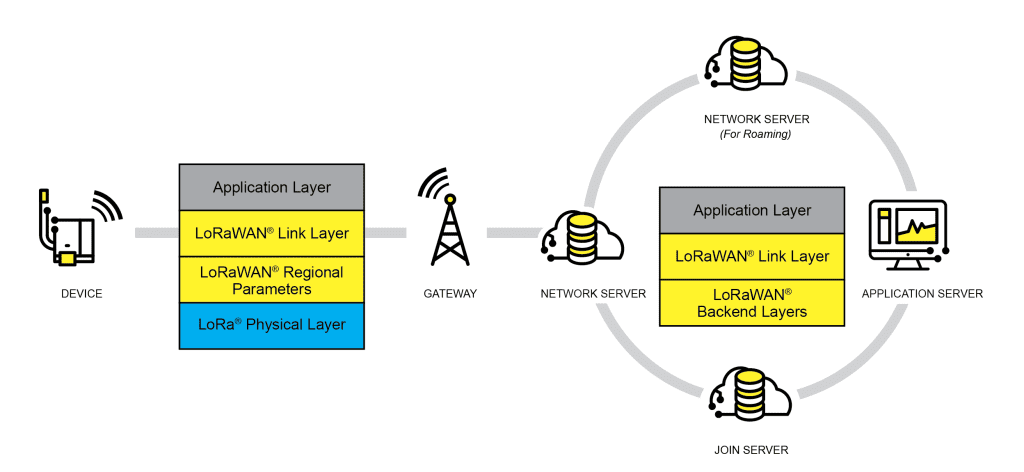
Attacker Identification In LoRaWAN Through Physical Channel Fingerprinting
LoRaWAN has recently emerged as one of the most important low-power wide area network (LPWAN) technologies due to its ability to combine long-distance communication with energy-efficient computations. LoRaWAN enables long-distance communication, allowing for new types of services in a variety of fields. There are several solutions available; LoRaWAN is arguably the most widely used. It…
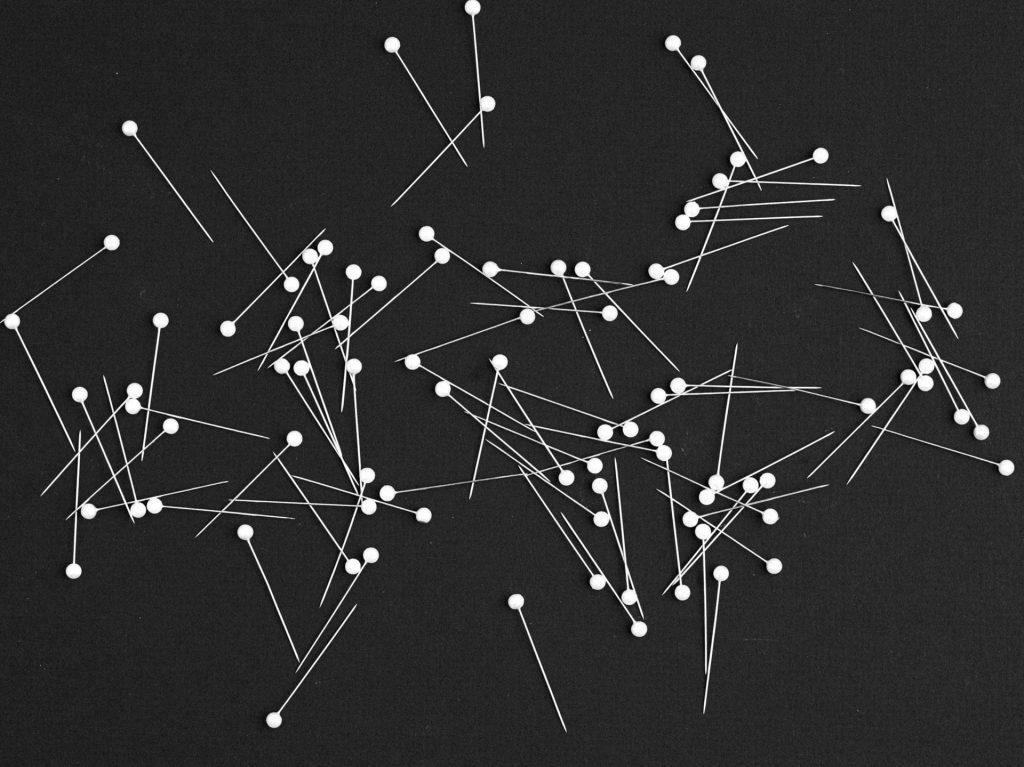
Clustering applications in Wireless Networks
Following this series of research articles, today we will briefly discuss some of our recent contributions of clustering applied to Wireless Networks. By definition, clustering consists of grouping a set of elements according to some criteria (often the similarity among these elements). We will start with a general review of the topic and then will…
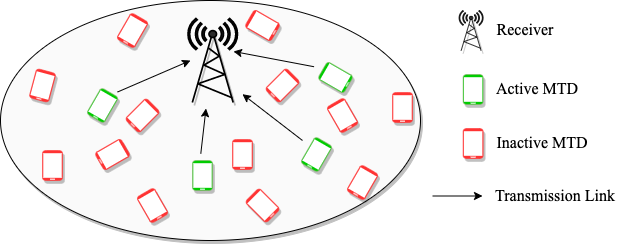
Multiagent RL and Scalability Challenges for Random Access in MTC
Machine-type communication (MTC) paradigm in wireless communication will help connect millions of devices to perform different tasks without human involvement. There are numerous use cases of MTC, such as a factory automation, fleet management, smart homes and smart metering, e-health and smart logistics, etc. Mostly, the devices called machine-type devices (MTDs) in the MTC network…
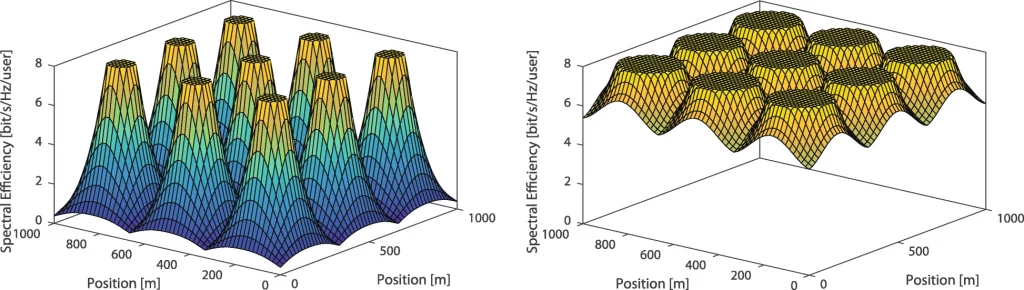
A short intro on Cell-Free Massive MIMO: Uniform Service for Everyone
The wireless communication community has proposed several different technologies to support increasing service demands from the first (1G) to fifth generation (5G). One obvious way to increase the network coverage and rate is densification: increasing the number of serving base stations (BSs) or increasing the number of antennas in each BS. In wireless communication terminology,…
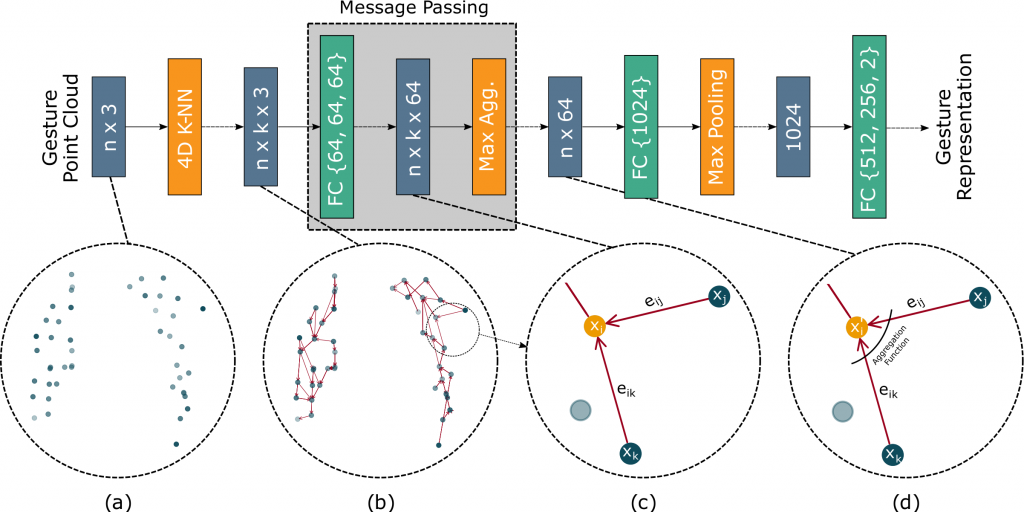
Zero-shot Motion Pattern Recognition from 4D point-clouds
Introduction In this post, we are going to discuss the pattern recognition from spatial data in motion through a zero-shot learning scenario. We use Siamese networks architecture to recognize unseen classes of motion patterns. A graph-based approach is adopted to achieve permutation invariance and encode moving point clouds into a representation in a computationally efficient way. Point-clouds…
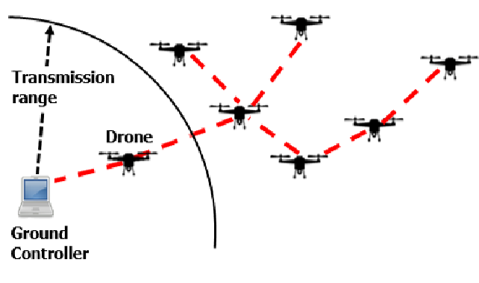
SMURF: Reliable Multipath Routing in Flying Ad-Hoc Networks
Unmanned Aerial Vehicles (UAVs) are used in a wide range of applications, from tracking and monitoring animals in remote areas [1] to military applications [2]. In general, drones are used to search, identify and monitor interesting events over massive and/or inaccessible areas. In order to effectively accomplish the task, multiple UAVs are deployed in a certain area and are expected to coordinate actions in an autonomous fashion or execute direct instructions from a control center.