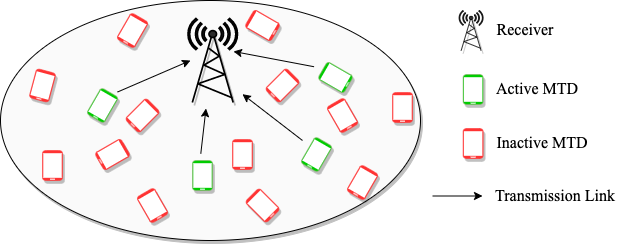
Multiagent RL and Scalability Challenges for Random Access in MTC
Machine-type communication (MTC) paradigm in wireless communication will help connect millions of devices to perform different tasks without human involvement. There are numerous use cases of MTC, such as a factory automation, fleet management, smart homes and smart metering, e-health and smart logistics, etc. Mostly, the devices called machine-type devices (MTDs) in the MTC network…
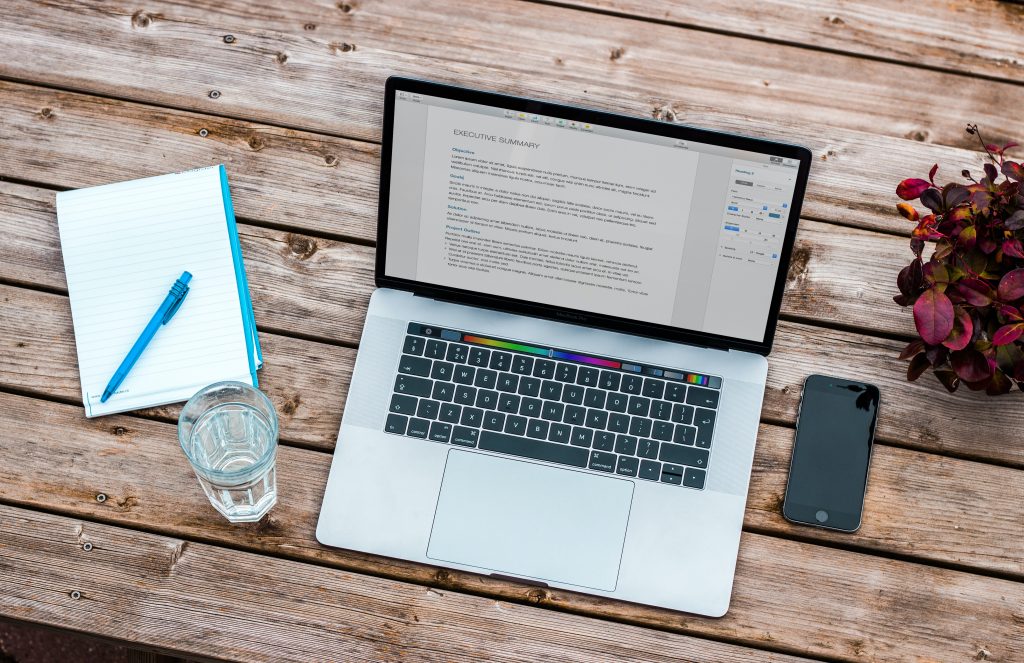
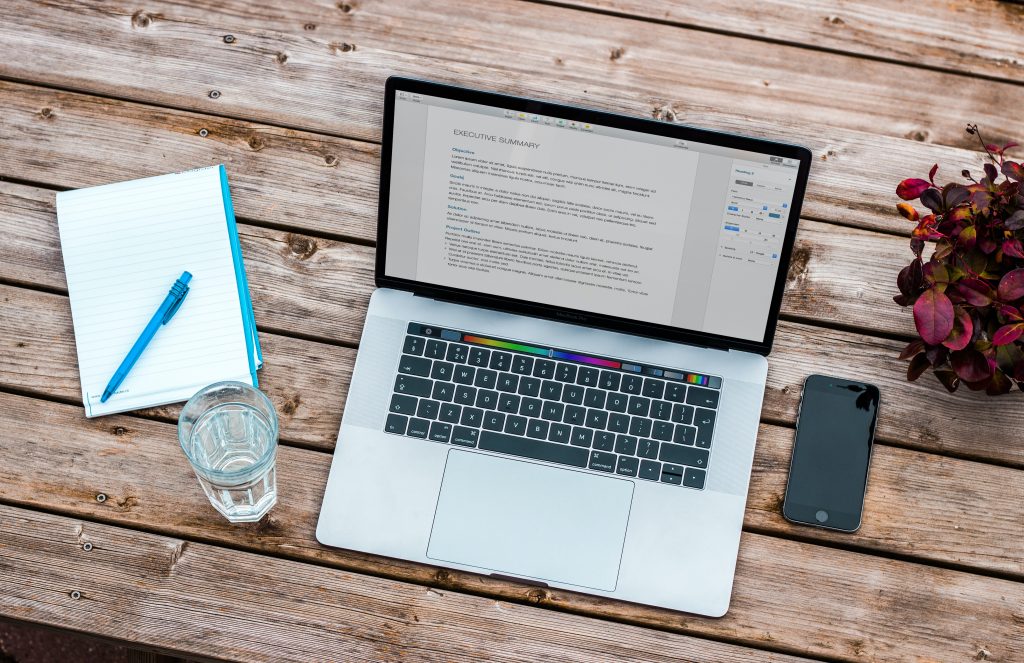
Online Courses for Studying ML
Hi everyone, I’m glad to meet all of you here again. If you are looking to start studying ML and have no idea how, especially considering the vast amount of information available on the web (which sometimes makes you feel completely lost), I can try clarifying it for you with today’s blog. I will recommend…
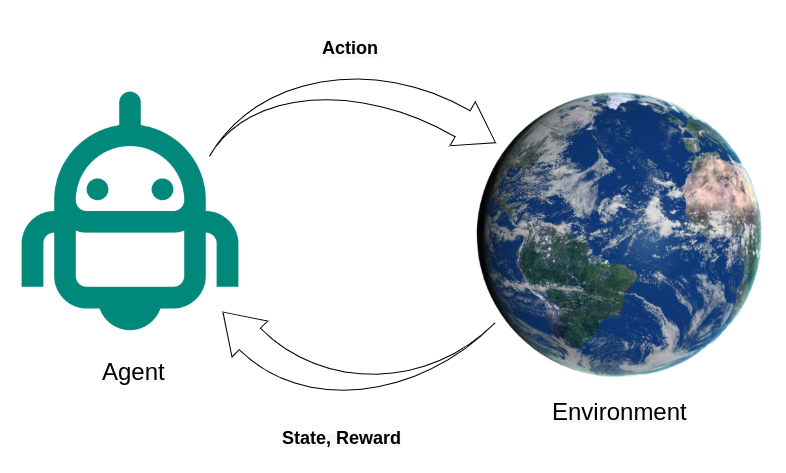
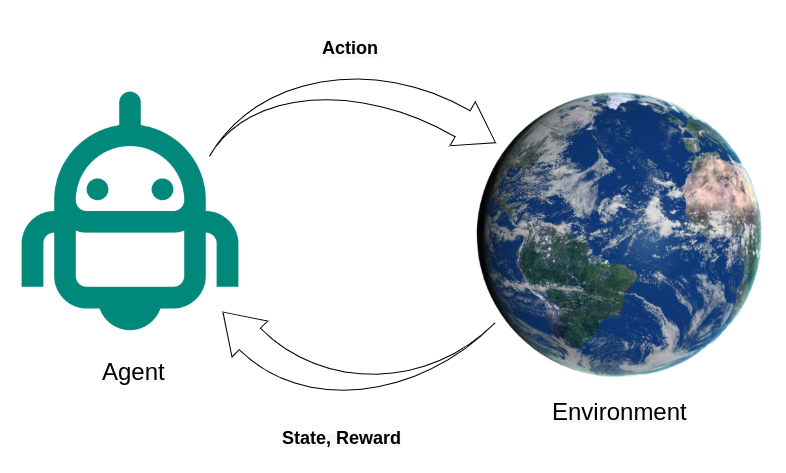
More humanoid agents with Hierarchical Reinforcement Learning
As you may have guessed from its name, Hierarchical Reinforcement Learning (HRL) is a family of Reinforcement Learning algorithms that decompose the problem into different hierarchies of subproblems or subtasks, and the higher-qlevel tasks invoke the primitive lower-level tasks. The goal of HRL is to learn a multilayer policy to perform control at different levels…
Slotted ALOHA with Reinforcement Learning
In this article we will discuss the potential of reinforcement learning (RL) to learn a backoff control policy for slotted ALOHA-type random access. We will use deep reinforcement learning (DRL) to learn a policy for multi user random access system. Slotted ALOHA Background Slotted ALOHA (sALOHA) protocol [1] for random access in wireless networks has…
Autonomous Vehicle Control using Reinforcement Learning
In this article, we are going to explore an application of an autonomous driving vehicle using reinforcement learning. First, we introduce the basic theory behind the control system of an autonomous ground vehicle (AGV). Then we simulate some AGV examples using an open source library denominated Python Robotics. Finally, we present how we can optimize…